Courses
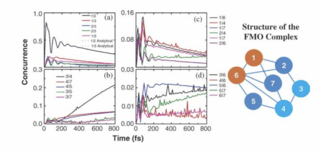
The development and use of quantum computers for chemical applications has the potential for revolutionary impact on the way computing is done in the future. Major challenge opportunities are abundant (see next fifteen chapters). One key example is developing and implementing quantum algorithms for solving chemical problems thought to be intractable for classical computers. Other challenges include the role of quantum entanglement, coherence, and superposition in photosynthesis and complex chemical reactions. Theoretical chemists have encountered and analyzed these quantum effects from the view of physical chemistry for decades. Therefore, combining results and insights from the quantum information community with those of the chemical physics community might lead to a fresh understanding of important chemical processes. In particular, we will discuss the role of entanglement in photosynthesis, in dissociation of molecules, and in the mechanism with which birds determine magnetic north. This chapter is intended to survey some of the most important recent results in quantum computation and quantum information, with potential applications in quantum chemistry. To start with, we give a comprehensive overview of the basics of quantum computing (the gate model), followed by introducing quantum simulation, where the phase estimation algorithm (PEA) plays a key role. Then we demonstrate how PEA combined with Hamiltonian simulation and multiplicative inversion can enable us to solve some types of linear systems of equations described by Ax = b. Then our subject turns from gate model quantum computing (GMQC) to adiabatic quantum computing (AQC) and topological quantum computing, which have gained increasing attention in the recent years due to their rapid progress in both theoretical and experimental areas. Finally, applications of the concepts of quantum information theory are usually related to the powerful and counter intuitive quantum mechanical effects of superposition, interference, and entanglement.
Introduction to Machine Learning and Quantum Computing (Prof. Batista)
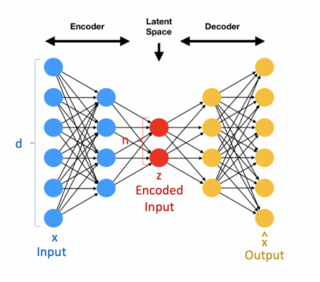
Machine learning and quantum computing have emerged as leading technologies of the twenty first century and are expected to be increasingly applied to address a wide variety of chemical and materials science challenges. The goal of this course is to introduce fundamental concepts of machine learning and quantum computing to chemists and materials science students through an overview of algorithms, computational methods, and applications. It is intended to empower students to engage with this emerging field and foster the growing field of artificial intelligence for accelerated scientific discoveries in the molecular and physical sciences.