Dissipative Quantum Dynamics of Energy Transfer with Unitary Circuits
Efficient energy transfer and dissipation is essential for suppressing recombination and stabilizing the products of photoinduced reactions. An outstanding challenge in the field is to establish quantum computing methods based on unitary circuits that could allow for simulations of dissipative reaction dynamics coupled to surrounding environments. Therefore, ongoing research efforts are focused on quantum mechanically exact methods based on generalized quantum master equations (GQMEs).

Proton Transfer Dynamics Simulated with Kerr-cat Quantum Devices
Our work explores driven Kerr-nonlinear resonators
for quantum reaction dynamics studies, enabling
research in areas hard to access through traditional
spectroscopy. This is useful for
examining proton/electron transfer dynamics,
highlighting the roles of quantum tunneling,
interference, and dissipation. We find that tunneling
in double-well models can lead to excited state
quantum phase transitions (ESQPTs) and that
reaction rates may defy Arrhenius predictions due to
a complex mix of resonances.
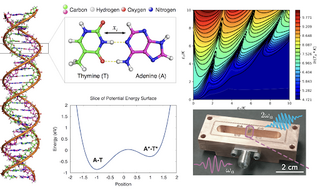
Mapping Molecular Hamiltonians and Propagators into Bosonic cQED Modular Devices
Bosonic modular devices have the possibility of simulations with hardware efficiency and shallow circuits. A question is how to map molecular systems of donor-acceptor systems into equal quantum circuits of modular quantum devices. An success of the CCI is the development of a general method based on the operators of the Dyson-Masleev transformation to map Hamiltonians, propagators, and dissipators of arbitrary model systems through parametrization of circuit Quantum Electrodynamics processors.
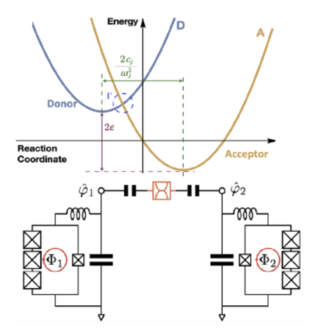
Boosting Amplitude Amplification in Variational Optimization for Molecular Systems
We have introduced a family of variational quantum algorithms, which we coined quantum iterative power algorithms (QIPAs) for global-optimization. We found that the QIPA based on a double exponential oracle can be efficiently applied to obtain the ground state of systems with fermions (e.g., molecules), or bosons (e.g., transmon), a method that outperforms quantum imaginary time evolution (QITE).
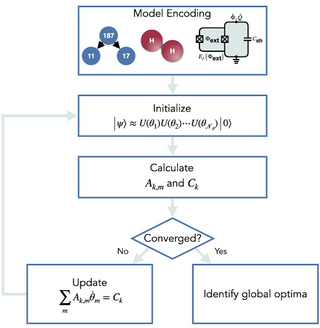
Holographic Quantum Computing Algorithms for Molecular Docking on 3D cQED Devices
We found that 3D cQED devices with a modest number of modes can be employed to simulate multimode systems with circuits that re-purpose working modes by iteratively applying two- or three-mode couplers, measurement and reinitialization. The process allows for holographic quantum computing in 3D cQED devices of multimode problems, including the implementation of Gaussian boson sampling algorithms applied for simulations of molecular docking.
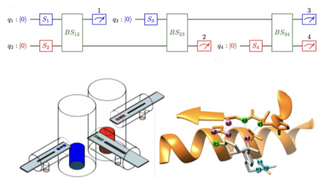
Quantum Search of Molecular Structures by Gaussian Boson Sampling
Quantum algorithms for solving the subgraph isomorphism problem can revolutionize drug design by identifying molecular structures efficiently. Gaussian Boson Sampling (GBS) offers a promising approach to this complex problem, simplifying the search for matching molecular substructures. Our method uses photonic technology to make this process scalable and flexible, potentially transforming molecular recognition and design in practical applications of pharmaceutical interest.
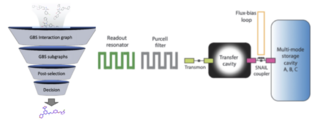
Hybrid Quantum-Classical Machine Learning Algorithms for Molecular Characterization
We have introduced quantum circuits to replace classical convolutional filters and develop hybrid quantum-classical neural networks for molecular classification tasks in applications to drug safety prediction. This work has introduced advanced quantum models based on quantum convolutional neural networks(QCNNs) that can process data with multiple channels. We envision the ability of the implementations will allow the implementation of quantum machine learning methods to operate in a wide range.
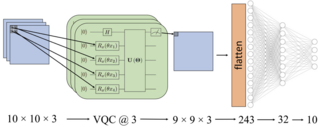
Mapping Molecular Electronic States to Photonic States
We have recently developed exact mapping methods to describe the electronic structure of molecules using photons in bosonic modular devices. This breakthrough could revolutionize how we simulate and understand complex molecular systems using quantum technology.
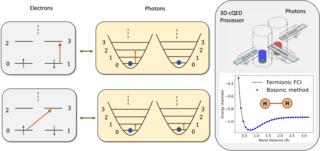
Quantum Computing Simulations of Conical Intersections
We focus on simulating realistic photo-triggered reaction dynamics using 3D cQED processors, parameterized with high-level theoretical methods. These simulations explore non-adiabatic processes at conical intersections, crucial points where quantum effects can influence chemical reactions. The intersections act as molecular “energy funnels,” guiding the reaction pathways. Similarly, our superconducting circuit design features a conical intersection, allowing us to model molecular processes.
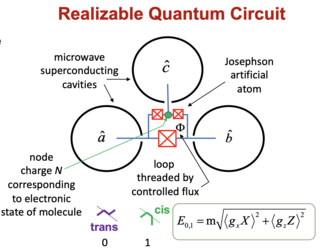
Quantum Kernel Autoencoder (KAE) Models for Generative Molecular Design
We are currently developing generative models based on quantum variational autoencoders of interest in a wide range of applications from de novo design of antibodies to retrosynthetic planning.
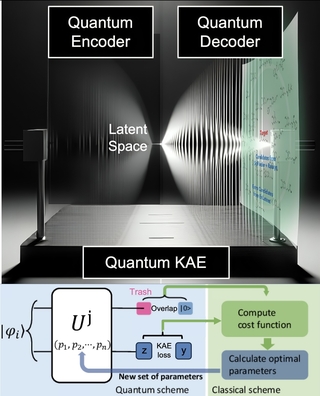
Electron-Transfer Dynamics in Organic Photovoltaics Simulated on NISQ Computers
Our recent quantum computing simulations have investigated how electron-transfer dynamics in an organic photovoltaic triad is boosted by coupling to the mode of a polaritonic cavity. The simulations demonstrate the capabilities of rigorous algorithms in realistic applications to model systems of technological interest.
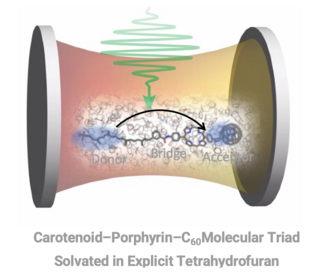